Featured Member
Imran Khan
Member
Imran Khan is a Professor in the Department of Pathology and Laboratory Medicine, University of California, Davis (UCD). He received his PhD from Albert Einstein College of Medicine, NY. His postdoctoral research, at UCD, studied the role of HIV gene products and their interactions with T cell signaling apparatus leading to AIDS pathogenesis, in the Rhesus monkey model. His research, as UCD faculty, has focused on infectious diseases and intracellular signaling in cancer cells, employing multiplex proteomic approaches. A major thrust has been on tuberculosis (TB) pathogenesis in monkey model and humans. He co-founded a biotechnology company, AppGenex Diagnostics Inc. (https://appgenex.com/), for the development of efficient diagnostics for infectious diseases, primarily TB. A disease of poverty, TB is the top infectious disease killer, and it has been on the rise for many decades, currently killing 1.6 million people worldwide, including about 50,000 in Pakistan, every year. This rise is surprising since anti-TB therapy is generally curative. But, being a complex disease there are limitations in its diagnosis. Imran’s research has led to the development of a novel TB blood test, currently under blinded trials at the Pakistan National Institutes of Health, and the Indian Council for Medical Research.
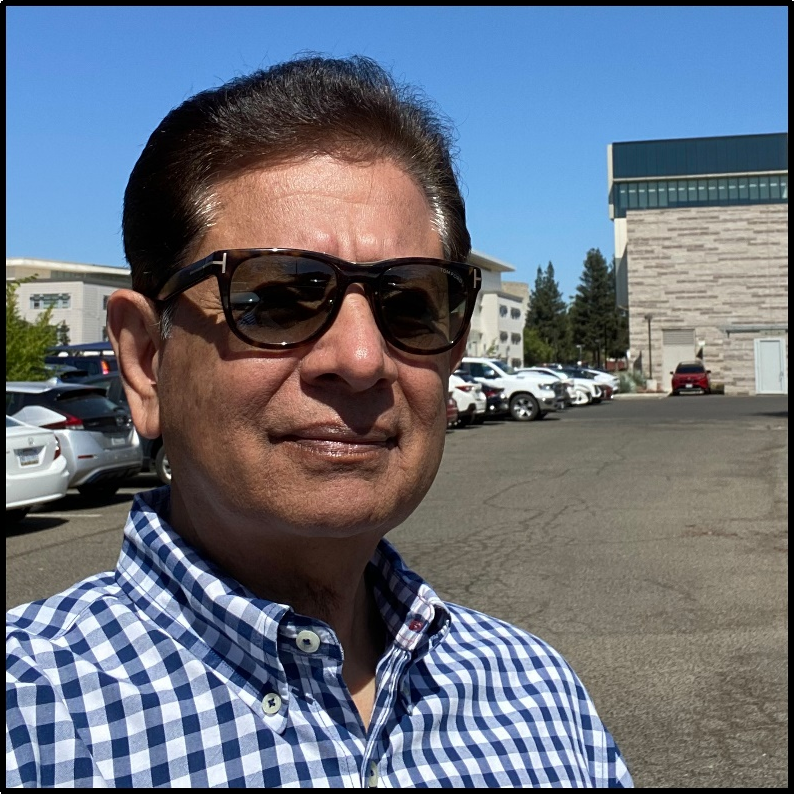
Publication
A key limitation in combating TB is inefficient detection of about 10 million new TB patients every year for which 5-10-fold as many TB-suspects need to be tested. This requires high-throughput sample analyses with automation capabilities. Imran’s laboratory seeks better understanding of disease pathogenesis to develop improved diagnostics. He and collaborators have used machine learning (ML) for high-throughput analysis of blood biomarkers data, and their integration with other diagnostic modalities, including radiologic imaging, to develop more accurate and comprehensive information for the physician.
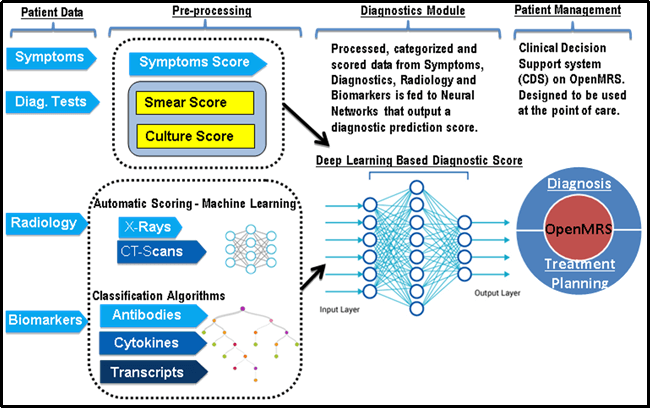
Legend: Multimodality TB diagnostics approach employing computational methods. Clinical and blood biomarkers data analyzed by Decision Tree algorithm, multimodal data integrated through Keras Machine Learning Systems, and the results delivered to the physician through Open-Medical Record System for Clinical Decision Support.
Rashidi HH, Dang LT, Albahra S, Ravindran R, and Khan IH. 2021. Automated Machine Learning for Endemic Active Tuberculosis Prediction from Multiplex Serological Data: Proof of Concept. 2021. Scientific Reports. https://doi.org/10.1038/s41598-021-97453-7.